Learning Journey on Informatics 2024
Keywords:
Decentralized Finance (DeFi) , Interoperability, Blockchain Networks , Security, Self-Supervised Learning, NoSQL, Differential Privacy, Social NetworksSynopsis
It is with pleasure that we present the 2024 edition of the book from the Learning Journey on Informatics series. The chapters in this book serve as supplementary material for the short courses selected to be presented at the Learning Journey on Informatics (JAI), traditionally held in conjunction with the Congress of the Brazilian Computing Society (SBC). The JAI is one of the most significant academic events for scientific and technological updates within the Brazilian computing community.
The JAI short courses cover current and relevant topics, are taught by experienced researchers, and provide an excellent opportunity for updating knowledge for academics and professionals in the field. This edition features 4 short courses presented during the SBC Congress. During the selection process, each proposal was reviewed by at least three evaluators, and the short course texts, which correspond to the chapters of this book, were reviewed by committee members to ensure their final quality.
Chapter 1 provides an in-depth discussion on three essential and driving aspects of the decentralized finance (DeFi) ecosystem: (1) innovative applications, (2) interoperability between blockchain networks, and (3) security for vulnerabilities in decentralized and competitive environments. In this context, the chapter also presents the fundamentals and practical examples of the most popular DeFi applications today, as well as discussing state-of-the-art research on the three aforementioned aspects that will support the growth of DeFi in the coming years.
Chapter 2 addresses and contextualizes self-supervised learning as an alternative for dynamic network applications, where data labeling is a critical challenge due to the discrepancy between traffic generation rates and manual data labeling rates. Generative and contrastive self-supervised learning techniques are presented for their effectiveness in improving network performance, expanding the number of labeled samples, and recognizing similarities and differences between sample examples. Finally, the chapter introduces self-supervised learning algorithms, their characteristics, and their applications in networks, aiming to equip readers with an understanding of the principles, frameworks, and limitations of this technique.
Chapter 3 presents the main concepts of NoSQL technology. Additionally, the chapter shows how to assess whether this technology is appropriate for the database design of a given system. The chapter also discusses some strategies for tuning the performance of NoSQL databases.
Chapter 4 provides an overview of differentially private methods and techniques to protect sensitive information while allowing relevant analysis of social networks. The principles of differential privacy are explored, highlighting mechanisms for adding noise to data to prevent individual reidentification. Additionally, strategies for applying differential privacy in social network data analysis are investigated, covering data publication, graph analysis, and machine learning tasks in a private manner.
We would like to thank the authors for submitting proposals and generating the final texts, and the Evaluation Committee for their dedication and efficiency throughout the short course selection process. Furthermore, we hope that all the material produced in this edition contributes to the education of students and professionals in the field.
Chapters
-
1. Decentralized Finance on Blockchain Networks: Perspectives on Research and Innovation in Applications, Interoperability, and Security
-
2. Generative and Contrastive Self-Supervised Learning: Trends and Challenges for Dynamic Network Applications
-
3. Design and Performance Tuning of NoSQL Databases
-
4. Private Data Analysis in Social Networks
Downloads
References
Abdulhamid, S. M., Ahmad, S., Waziri, V. O., and Jibril, F. N. (2014). Privacy and national security issues in social networks: the challenges. arXiv preprint arXiv:1402.3301.
Abusitta, A., de Carvalho, G. H., Wahab, O. A., Halabi, T., Fung, B. C. e Mamoori, S. A. (2023). Deep learning-enabled anomaly detection for iot systems. Internet of Things, 21:100656.
Acharya, J., Sun, Z., and Zhang, H. (2019). Hadamard response: Estimating distributions privately, efficiently, and with little communication. In The 22nd International Conference on Artificial Intelligence and Statistics, pages 1120–1129. PMLR.
Allardyce, C. S. (2012). Fat chemistry: The science behind obesity. Royal Society of Chemistry.
Allen, F., Gu, X., and Jagtiani, J. (2022). Fintech, cryptocurrencies, and cbdc: Financial structural transformation in china. Journal of International Money and Finance, 124:102625.
Almakhour, M., Sliman, L., Samhat, A. E., and Mellouk, A. (2020). Verification of smart contracts: A survey. Pervasive and Mobile Computing, 67:101227.
Alsmadi, I. and Alhami, I. (2015). Clustering and classification of email contents. Journal of King Saud University-Computer and Information Sciences, 27(1):46–57.
Ambler, S. W. (2000). Mapping objects to relational databases. White Paper, Ronin International.
Androulaki, E., Barger, A., Bortnikov, V., Cachin, C., Christidis, K., De Caro, A., Enyeart, D., Ferris, C., Laventman, G., Manevich, Y., et al. (2018). Hyperledger fabric: a distributed operating system for permissioned blockchains. In Proceedings of the thirteenth EuroSys conference, pages 1–15.
Bachman, P., Hjelm, R. D. e Buchwalter, W. (2019). Learning representations by maximizing mutual information across views. Advances in neural information processing systems, 32.
Back, A., Corallo, M., Dashjr, L., Friedenbach, M., Maxwell, G., Miller, A., Poelstra, A., Timón, J., and Wuille, P. (2014). Enabling blockchain innovations with pegged sidechains. URL: [link], 72:201–224.
Baden, R., Bender, A., Spring, N., Bhattacharjee, B., and Starin, D. (2009). Persona: an online social network with user-defined privacy. In Proceedings of the ACM SIGCOMM 2009 conference on Data communication, pages 135–146.
Barbosa, G. N. N., Andreoni Lopez, M., Medeiros, D. S. V. e Mattos, D. M. F. (2021a). An entropy-based hybrid mechanism for large-scale wireless network traffic prediction. Em 2021 International Symposium on Networks, Computers and Communications (ISNCC), p. 1–6.
Barbosa, G. N. N., Andreoni, M. e Mattos, D. M. F. (2024). Optimizing feature selection in intrusion detection systems: Pareto dominance set approaches with mutual information and linear correlation. Ad Hoc Networks, 159:103485.
Barbosa, G. N. N., Bezerra, G. M. G., de Medeiros, D. S. V., Andreoni Lopez, M. e Mattos, D. M. F. (2021b). Segurança em Redes 5G: Oportunidades e Desafios em Detecção de Anomalias e Predição de Tráfego baseadas em Aprendizado de Máquina. Em Minicursos do XXI Simpósio Brasileiro de Segurança da Informação e de Sistemas Computacionais, p. 145–189.
Bardes, A., Ponce, J. e LeCun, Y. (2022). Vicreg: Varianceinvariance- covariance regularization for self-supervised learning. ICLR, Vicreg, 1:2.
Bassily, R. and Smith, A. (2015). Local, private, efficient protocols for succinct histograms. In Proceedings of the forty-seventh annual ACM symposium on Theory of computing, pages 127–135.
Beillahi, S. M., Keilty, E., Nelaturu, K., Veneris, A., and Long, F. (2022). Automated auditing of price gouging tod vulnerabilities in smart contracts. In 2022 IEEE International Conference on Blockchain and Cryptocurrency (ICBC), pages 1–6. IEEE.
Belchior, R., Vasconcelos, A., Guerreiro, S., and Correia, M. (2021). A survey on blockchain interoperability: Past, present, and future trends. ACM Computing Surveys (CSUR), 54(8):1–41.
Benymol, J. and Sajimon, A. (2017). Exploring the merits of nosql: A study based on mongodb. International Conference on Networks & Advances in Computational Technologies (NetACT), pages 20–22.
Berman, P. and Garay, J. A. (1993). Cloture votes: n/4- resilient distributed consensus in t+ 1 rounds. Mathematical systems theory, 26:3–19.
Bernstein, P. A. and Goodman, N. (1981). Concurrency control in distributed database systems. ACM Comput. Surv., 13(2):185–221.
Bernstein, P. A., Hadzilacos, V., and Goodman, N. (1987). Concurrency Control and Recovery in Database Systems. Addison-Wesley.
Besançon, L., Silva, C. F. D., and Ghodous, P. (2019). Towards blockchain interoperability: Improving video games data exchange. In 2019 IEEE International Conference on Blockchain and Cryptocurrency (ICBC), pages 81–85.
Bessani, A., Sousa, J., and Alchieri, E. E. (2014). State machine replication for the masses with bft-smart. In 2014 44th Annual IEEE/IFIP International Conference on Dependable Systems and Networks, pages 355–362. IEEE.
Bhaila, K., Huang, W., Wu, Y., and Wu, X. (2023). Local differential privacy in graph neural networks: a reconstruction approach. arXiv preprint arXiv:2309.08569.
Binance (2023). O que é uma stablecoin? [link]. (Accessed on 05/23/2024).
Bloch, F., Jackson, M. O., and Tebaldi, P. (2023). Centrality measures in networks. Social Choice and Welfare, 61(2):413–453.
Bochie, K., da Silva Gilbert, M., Gantert, L., Barbosa, M. d. S. M., de Medeiros, D. S. V. e Campista, M. E. M. (2020). Aprendizado profundo em redes desafiadoras: Conceitos e aplicações. Sociedade Brasileira de Computação.
Bondi, A. B. (2000). Characteristics of scalability and their impact on performance. In Second International Workshop on Software and Performance, WOSP 2000, Ottawa, Canada, September 17-20, 2000, pages 195–203. ACM.
Boscarioli, C., Bezerra, A., BENEDICTO, M. d., and Delmiro, G. (2006). Uma reflexão sobre banco de dados orientados a objetos. In Congresso de Tecnologias para Gestão de Dados e Metadados do Cone Sul, Paraná, Brasil. sn.
Boudaoud, A., Mahfoud, H., and Chikh, A. (2022). Towards a complete direct mapping from relational databases to property graphs. In Fournier-Viger, P., Yousef, A. H., and Bellatreche, L., editors, Model and Data Engineering: 11th International Conference, MEDI 2022, Cairo, Egypt, November 21-24, 2022, Proceedings, volume 13761 of Lecture Notes in Computer Science, pages 222–235. Springer.
Boyd, D. M. and Ellison, N. B. (2007). Social network sites: Definition, history, and scholarship. Journal of computer-mediated Communication, 13(1):210–230.
Brazil (2018). Lei Geral de Proteção de Dados Pessoais. [link]. Acesso em: 22 de maio de 2024.
Breidenbach, L., Cachin, C., Chan, B., Coventry, A., Ellis, S., Juels, A., Koushanfar, F., Miller, A., Magauran, B., Moroz, D., et al. (2022). Chainlink 2.0: Next steps in the evolution of decentralized oracle networks. 2021. Available also from: [link].
Brent, L., Jurisevic, A., Kong, M., Liu, E., Gauthier, F., Gramoli, V., Holz, R., and Scholz, B. (2018). Vandal: A scalable security analysis framework for smart contracts. arXiv preprint arXiv:1809.03981.
Brito, F. T. (2023). Differentially private release of count-weighted graphs. PhD thesis, Universidade Federal do Ceará.
Brito, F. T. and Machado, J. C. (2017). Preservação de privacidade de dados: Fundamentos, técnicas e aplicações. Jornadas de atualização em informática, pages 91–130.
Brito, F. T., Farias, V. A., Flynn, C., Majumdar, S., Machado, J. C., and Srivastava, D. (2023). Global and local differentially private release of count-weighted graphs. Proceedings of the ACM on Management of Data, 1(2):1–25.
Brito, F. T., Mendonça, A. L. C., and Machado, J. C. (2024). A differentially private guide for graph analytics. In Proceedings 27th International Conference on Extending Database Technology, EDBT 2024, Paestum, Italy, March 25 - March 28, pages 850–853. OpenProceedings.org.
Brito, F. T., Neto, A. C. A., Costa, C. F., Mendonça, A. L., and Machado, J. C. (2015). A distributed approach for privacy preservation in the publication of trajectory data. In Proceedings of the 2nd Workshop on Privacy in Geographic Information Collection and Analysis, pages 1–8.
Burrows, M. (2006). The chubby lock service for loosely-coupled distributed systems. In Proceedings of the 7th symposium on Operating systems design and implementation, pages 335–350.
Buterin, V. (2016). Chain interoperability. R3 research paper, 9:1–25.
Buterin, V. et al. (2014). A next-generation smart contract and decentralized application platform. white paper, 3(37):2–1.
Caldarelli, G. and Ellul, J. (2021). The blockchain oracle problem in decentralized finance—a multivocal approach. Applied Sciences, 11(16):7572.
Cao, Y., Zou, C., and Cheng, X. (2021). Flashot: a snapshot of flash loan attack on defi ecosystem. arXiv preprint arXiv:2102.00626.
Caron, M., Misra, I., Mairal, J., Goyal, P. e Bojanowski, P. (2020). Unsupervised learning of visual features by contrasting cluster assignments. Em European Conference on Computer Vision, p. 3–19.
Caron, M., Sun, R. e Schölkopf, B. (2018). Counterfactuals uncover the modular structure of deep generative models. arXiv preprint arXiv:1812.03253.
Caron, M., Touvron, H., Misra, I., Jégou, H., Mairal, J., Bojanowski, P. e Joulin, A. (2021). Emerging properties in self-supervised vision transformers. Em Proceedings of the IEEE/CVF international conference on computer vision, p. 9650–9660.
Casino, F., Dasaklis, T. K., and Patsakis, C. (2019). A systematic literature review of blockchain-based applications: Current status, classification and open issues. Telematics and informatics, 36:55–81.
Cassandra Database (2024). Open Source Nosql Database. Disponível em: [link]. Acessado em: 01/05/2024.
Cassandra Documentation (2024). Welcome to apache cassandras documentation! Disponível em: [link]. Acessado em: 01/05/2024.
Chaabane, A., Acs, G., Kaafar, M. A., et al. (2012). You are what you like! information leakage through users’ interests. In Proceedings of the 19th annual network & distributed system security symposium (NDSS). Citeseer.
Chaliasos, S., Charalambous, M. A., Zhou, L., Galanopoulou, R., Gervais, A., Mitropoulos, D., and Livshits, B. (2024). Smart contract and defi security tools: Do they meet the needs of practitioners? In Proceedings of the 46th IEEE/ACM International Conference on Software Engineering, pages 1–13.
Chandra, T. D., Griesemer, R., and Redstone, J. (2007). Paxos made live: an engineering perspective. In Proceedings of the twenty-sixth annual ACM symposium on Principles of distributed computing, pages 398–407.
Chen, L., Han, K., Xiu, Q., and Gao, D. (2022). Graph clustering under weight-differential privacy. In 2022 IEEE 24th Int Conf on High Performance Computing & Communications; 8th Int Conf on Data Science & Systems; 20th Int Conf on Smart City; 8th Int Conf on Dependability in Sensor, Cloud & Big Data Systems & Application (HPCC/DSS/SmartCity/DependSys), pages 1457–1464. IEEE.
Chen, R., Fung, B., Yu, P. S., and Desai, B. C. (2014). Correlated network data publication via differential privacy. The VLDB Journal, 23(4):653–676.
Chen, T., He, X., Fan, Y., Zhang, Y. e Xie, J. (2020a). Exploring simple siamese representation learning. arXiv preprint arXiv:2011.10566.
Chen, T., Kornblith, S., Norouzi, M. e Hinton, G. (2020b). A simple framework for contrastive learning of visual representations. Em International conference on machine learning, p. 1597–1607. PMLR.
Chen, X. e He, K. (2021). Exploring simple siamese representation learning. Em Proceedings of the IEEE/CVF conference on computer vision and pattern recognition, p. 15750–15758.
Chen, Y., Richter, J. I., and Patel, P. C. (2021). Decentralized governance of digital platforms. Journal of Management, 47(5):1305–1337.
Chohan, U.W. (2021). The double spending problem and cryptocurrencies. Available at SSRN 3090174.
Choi, J. e Ryu, D. (2023). Qos-aware graph contrastive learning for web service recommendation. Em 2023 30th Asia-Pacific Software Engineering Conference (APSEC), p. 171–180. IEEE.
Clauset, A., Moore, C., and Newman, M. E. (2006). Structural inference of hierarchies in networks. In ICML Workshop on Statistical Network Analysis, pages 1–13. Springer.
ConsenSys (2024). Mythril: A security analysis tool for evm bytecode.
Conti, M., Kumar, E. S., Lal, C., and Ruj, S. (2018). A survey on security and privacy issues of bitcoin. IEEE communications surveys & tutorials, 20(4):3416–3452.
Cook, D. J. and Holder, L. B. (2006). Mining graph data. John Wiley & Sons.
Cormode, G., Jha, S., Kulkarni, T., Li, N., Srivastava, D., and Wang, T. (2018). Privacy at scale: Local differential privacy in practice. In Proceedings of the 2018 International Conference on Management of Data, pages 1655–1658.
Cormode, G., Procopiuc, C., Srivastava, D., and Tran, T. T. (2012). Differentially private summaries for sparse data. In Proceedings of the 15th International Conference on Database Theory, pages 299–311.
Costa, D., La Cava, L., and Tagarelli, A. (2023). Show me your nft and i tell you how it will perform: Multimodal representation learning for nft selling price prediction. In Proceedings of the ACM Web Conference 2023, pages 1875–1885.
Creswell, A., White, T., Dumoulin, V., Arulkumaran, K., Sengupta, B. e Bharath, A. A. (2018). Generative adversarial networks: An overview. IEEE Signal Processing Magazine, 35(1):53–65.
da Costa Filho, J. S. and Machado, J. C. (2023). FELIP: A local differentially private approach to frequency estimation on multidimensional datasets. In Proceedings 26th International Conference on Extending Database Technology, EDBT 2023, Ioannina, Greece, March 28-31, 2023, pages 671–683. Open- Proceedings.org.
Dai, Z., Yang, Z., Yang, Y., Carbonell, J., Le, Q. V. e Salakhutdinov, R. (2019). Transformer-xl: Attentive language models beyond a fixed-length context. arXiv preprint arXiv:1901.02860.
Daigavane, A., Madan, G., Sinha, A., Thakurta, A. G., Aggarwal, G., and Jain, P. (2021). Node-level differentially private graph neural networks. ar- Xiv:2111.15521.
Dalenius, T. (1977). Towards a methodology for statistical disclosure control.
David, I., Zhou, L., Qin, K., Song, D., Cavallaro, L., and Gervais, A. (2023). Do you still need a manual smart contract audit? arXiv preprint ar- Xiv:2306.12338.
Davoudian, A., Chen, L., and Liu, M. (2018). A survey on nosql stores. ACM Comput. Surv., 51(2):40:1–40:43.
Day,W.-Y., Li, N., and Lyu, M. (2016). Publishing graph degree distribution with node differential privacy. In Proceedings of the 2016 International Conference on Management of Data, pages 123–138.
de Oliveira, N. R., Pisa, P. S., Andreoni Lopez, M., de Medeiros, D. S. V. e Mattos, D. M. F. (2021). Identifying fake news on social networks based on natural language processing: Trends and challenges. Information, 12(1).
Deng, J., Dong, W., Socher, R., Li, L.-J., Li, K. e Fei-Fei, L. (2009). ImageNet: A Large-Scale Hierarchical Image Database. Em CVPR09.
Deshpande, A. and Herlihy, M. (2020). Privacy-preserving cross-chain atomic swaps. In International Conference on Financial Cryptography and Data Security, pages 540–549. Springer.
Devlin, J., Chang, M.-W., Lee, K. e Toutanova, K. (2018). Bert: Pretraining of deep bidirectional transformers for language understanding. arXiv preprint arXiv:1810.04805.
Dey, R., Tang, C., Ross, K., and Saxena, N. (2012). Estimating age privacy leakage in online social networks. In 2012 proceedings ieee infocom, pages 2836–2840. IEEE.
Dib, M., Torabi, S., Bou-Harb, E., Bouguila, N. e Assi, C. (2022). Evoliot: A self-supervised contrastive learning framework for detecting and characterizing evolving iot malware variants. Em Proceedings of the 2022 ACM on Asia Conference on Computer and Communications Security, p. 452–466.
Ding, M., Li, P., Li, S., and Zhang, H. (2021). Hfcontractfuzzer: Fuzzing hyperledger fabric smart contracts for vulnerability detection. In Proceedings of the 25th International Conference on Evaluation and Assessment in Software Engineering, pages 321–328.
Doersch, C., Gupta, A. e Efros, A. A. (2015). Unsupervised visual representation learning by context prediction. Em Proceedings of the IEEE international conference on computer vision, p. 1422–1430.
Duchi, J. C., Jordan, M. I., and Wainwright, M. J. (2013). Local privacy and statistical minimax rates. In 2013 IEEE 54th Annual Symposium on Foundations of Computer Science, pages 429–438. IEEE.
Dwork, C. (2006). Differential privacy. In International Colloquium on Automata, Languages, and Programming, pages 1–12. Springer.
Dwork, C., McSherry, F., Nissim, K., and Smith, A. (2006). Calibrating noise to sensitivity in private data analysis. In Theory of cryptography conference, pages 265–284. Springer.
Dwork, C., Roth, A., et al. (2014). The algorithmic foundations of differential privacy. Foundations and Trends® in Theoretical Computer Science, 9(3–4):211–407.
Elmasri, R. and Navathe, S. B. (2000). Fundamentals of Database Systems, 3rd Edition. Addison-Wesley-Longman.
Entriken,W., Shirley, D., Evans, J., and Sachs, N. (2018). Ethereum improvement proposal 721.
Erlingsson, Ú., Pihur, V., and Korolova, A. (2014). Rappor: Randomized aggregatable privacy-preserving ordinal response. In Proceedings of the 2014 ACM SIGSAC conference on computer and communications security, pages 1054–1067. ACM.
Ermolov, A., Siarohin, A., Sangineto, E. e Sebe, N. (2021). Whitening for self-supervised representation learning. Em International conference on machine learning, p. 3015–3024. PMLR.
Eskandari, S., Moosavi, S., and Clark, J. (2020). Sok: Transparent dishonesty: front-running attacks on blockchain. In Financial Cryptography and Data Security: FC 2019 International Workshops, VOTING and WTSC, St. Kitts, St. Kitts and Nevis, February 18–22, 2019, Revised Selected Papers 23, pages 170–189. Springer.
European Commission (2018). 2018 reform of EU data protection rules. [link]. Acesso em: 22 de maio de 2024.
Faerber, F., Kemper, A., Larson, P., Levandoski, J. J., Neumann, T., and Pavlo, A. (2017). Main memory database systems. Foundations and Trends in Databases, 8(1-2):1–130.
Fan, C. and Li, P. (2022). Distances release with differential privacy in tree and grid graph. In 2022 IEEE International Symposium on Information Theory (ISIT), pages 2190–2195. IEEE.
Farias, V. A., Brito, F. T., Flynn, C., Machado, J. C., Majumdar, S., and Srivastava, D. (2020). Local dampening: differential privacy for non-numeric queries via local sensitivity. Proceedings of the VLDB Endowment, 14(4):521–533.
Farias, V. A., Brito, F. T., Flynn, C., Machado, J. C., Majumdar, S., and Srivastava, D. (2023). Local dampening: Differential privacy for non-numeric queries via local sensitivity. The VLDB Journal, pages 1–24.
Frost, J., Gambacorta, L., Huang, Y., Shin, H. S., and Zbinden, P. (2019). Bigtech and the changing structure of financial intermediation. Economic policy, 34(100):761–799.
Frozza, A. A., Schreiner, G. A., and dos Santos Mello, R. (2022). Projeto de bancos de dados nosql. 37th Brazilian Symposium on Data Bases.
Gao, Y., Hasegawa, H., Yamaguchi, Y. e Shimada, H. (2022). Unsupervised graph contrastive learning with data augmentation for malware classification. Em Proc. 16th International Conference on Emerging Security Information, Systems and Technologies (SECURWARE 2022), IARIA, p. 41–47.
Garfinkel, S. L., Abowd, J. M., and Powazek, S. (2018). Issues encountered deploying differential privacy. In Proceedings of the 2018 Workshop on Privacy in the Electronic Society, pages 133–137. ACM.
Germain, M., Gregor, K., Murray, I. e Larochelle, H. (2015). Made: Masked autoencoder for distribution estimation. International Conference on Machine Learning, p. 881–889.
Ghaleb, A., Rubin, J., and Pattabiraman, K. (2023). Achecker: Statically detecting smart contract access control vulnerabilities. In 2023 IEEE/ACM 45th International Conference on Software Engineering (ICSE), pages 945–956. IEEE.
Ghosh, A., Roughgarden, T., and Sundararajan, M. (2009). Universally utility-maximizing privacy mechanisms. In Proceedings of the forty-first annual ACM symposium on Theory of computing, pages 351–360.
Gidaris, S., Singh, P. e Komodakis, N. (2018). Unsupervised representation learning by predicting image rotations. arXiv preprint arXiv:1803.07728.
Gilbert, S. and Lynch, N. (2002). Brewer’s conjecture and the feasibility of consistent, available, partition-tolerant web services. Acm Sigact News, 33(2):51–59.
Goes, C. (2020). The interblockchain communication protocol: An overview. arXiv preprint arXiv:2006.15918.
Goetze, C. (2023). Stablecoins: o que são e quais os tipos? [link]. (Accessed on 05/23/2024).
Gong, N. Z., Talwalkar, A., Mackey, L., Huang, L., Shin, E. C. R., Stefanov, E., Shi, E., and Song, D. (2014). Joint link prediction and attribute inference using a social-attribute network. ACM Transactions on Intelligent Systems and Technology (TIST), 5(2):1–20.
González, G. G., Casas, P. e Fernández, A. (2023). Fake it till you detect it: Continual anomaly detection in multivariate time-series using generative ai. Em 2023 IEEE European Symposium on Security and Privacy Workshops (EuroS& PW), p. 558–566. IEEE.
Goyal, P., Caron, M., Lefaudeux, B., Xu, M., Wang, P., Pai, V., Singh, M., Liptchinsky, V., Misra, I., Joulin, A. et al. (2021). Self-supervised pretraining of visual features in the wild. arXiv preprint arXiv:2103.01988.
Gray, J. and Reuter, A. (1993). Transaction Processing: Concepts and Techniques. Morgan Kaufmann.
Gray, J., Lorie, R. A., Putzolu, G. R., and Traiger, I. L. (1975). Granularity of locks in a large shared data base. In Kerr, D. S., editor, Proceedings of the International Conference on Very Large Data Bases, September 22-24, 1975, Framingham, Massachusetts, USA, pages 428–451. ACM.
Greve, F., Sampaio, L., Abijaude, J., Coutinho, A. A., Brito, I., and Queiroz, S. (2018). Blockchain e a Revolução do Consenso sob Demanda. In Proc. of SBRC Minicursos.
Grill, J.-B., Strub, F., Altche, F., Tallec, C. L. e Richemond, P. H. (2020). Bootstrap your own latent: A new approach to self-supervised learning. Em Advances in Neural Information Processing Systems, p. 33–44.
Gueidi, A., Gharsellaoui, H., and Ahmed, S. B. (2021). Towards unified modeling for nosql solution based on mapping approach. In Watróbski, J., Salabun, W., Toro, C., Zanni-Merk, C., Howlett, R. J., and Jain, L. C., editors, Knowledge-Based and Intelligent Information & Engineering Systems: Proceedings of the 25th International Conference KES-2021, Virtual Event / Szczecin, Poland, 8-10 September 2021, volume 192 of Procedia Computer Science, pages 3637–3646. Elsevier.
Gutmann, M. e Hyvärinen, A. (2010). Noise-contrastive estimation: A new estimation principle for unnormalized statistical models. Em Proceedings of the thirteenth international conference on artificial intelligence and statistics, p. 297–304. JMLR Workshop and Conference Proceedings.
Han, X., Cui, S., Qin, J., Liu, S., Jiang, B., Dong, C., Lu, Z. e Liu, B. (2024). Contramtd: An unsupervised malicious network traffic detection method based on contrastive learning. Em Proceedings of the ACM on Web Conference 2024, p. 1680–1689.
Härder, T. and Reuter, A. (1983). Principles of transaction-oriented database recovery. ACM Comput. Surv., 15(4):287–317.
Harrison, G. (2015). Next Generation Databases: NoSQLand Big Data. Apress Berkeley, CA.
Harvey, C. R., Ramachandran, A., and Santoro, J. (2021). DeFi and the Future of Finance. John Wiley & Sons.
Harz, D. and Knottenbelt, W. (2018). Towards safer smart contracts: A survey of languages and verification methods. arXiv preprint ar- Xiv:1809.09805.
Hassan, S. and De Filippi, P. (2021). Decentralized autonomous organization. Internet Policy Review, 10(2):1–10.
Hassani, K. e Khasahmadi, A. H. (2020). Contrastive multi-view representation learning on graphs. Em International conference on machine learning, p. 4116–4126. PMLR.
Hay, M., Li, C., Miklau, G., and Jensen, D. (2009). Accurate estimation of the degree distribution of private networks. In 2009 Ninth IEEE International Conference on Data Mining, pages 169–178. IEEE.
He, K., Fan, H., Wu, Y., Xie, S. e Girshick, R. (2020a). Momentum contrast for unsupervised visual representation learning. Em Proceedings of the IEEE/CVF Conference on Computer Vision and Pattern Recognition, p. 9729–9738.
He, K., Fan, H., Wu, Y., Xie, S. e Girshick, R. (2020b). Momentum contrast for unsupervised visual representation learning. Em Proceedings of the IEEE/CVF Conference on Computer Vision and Pattern Recognition, p. 9729–973.
Helliar, C. V., Crawford, L., Rocca, L., Teodori, C., and Veneziani, M. (2020). Permissionless and permissioned blockchain diffusion. International Journal of Information Management, 54:102136.
Heuser, C. A. (2009). Projeto de banco de dados: Volume 4 da Série Livros didáticos informática UFRGS. Bookman Editora.
Hill, M. D. (1990). What is scalability? SIGARCH Comput. Archit. News, 18(4):18–21.
Hsu, J., Gaboardi, M., Haeberlen, A., Khanna, S., Narayan, A., Pierce, B. C., and Roth, A. (2014). Differential privacy: An economic method for choosing epsilon. In 2014 IEEE 27th Computer Security Foundations Symposium, pages 398–410. IEEE.
Iftikhar, M., Wang, Q., and Lin, Y. (2020). dk-microaggregation: Anonymizing graphs with differential privacy guarantees. In Pacific-Asia Conference on Knowledge Discovery and Data Mining, pages 191–203. Springer.
Imielinski, T. and Jr., W. L. (1984). The relational model of data and cylindric algebras. J. Comput. Syst. Sci., 28(1):80–102.
Imola, J., Murakami, T., and Chaudhuri, K. (2021). Locally differentially private analysis of graph statistics. In 30th USENIX Security Symposium (USENIX Security 21), pages 983–1000.
Ivanov, N., Li, C., Yan, Q., Sun, Z., Cao, Z., and Luo, X. (2023). Security threat mitigation for smart contracts: A comprehensive survey. ACM Computing Surveys, 55(14s):1–37.
Jaiswal, A., Babu, A. R., Zadeh, M. Z., Banerjee, D. e Makedon, F. (2021). A survey on contrastive self-supervised learning. Technologies, 9(1).
Ji, T., Li, P., Yilmaz, E., Ayday, E., Ye, Y., and Sun, J. (2021). Differentially private binary-and matrix-valued data query: an xor mechanism. Proceedings of the VLDB Endowment, 14(5):849–862.
Ji, T., Luo, C., Guo, Y., Ji, J., Liao, W., and Li, P. (2019). Differentially private community detection in attributed social networks. In Asian Conference on Machine Learning, pages 16–31. PMLR.
Jian, X., Wang, Y., and Chen, L. (2021). Publishing graphs under node differential privacy. IEEE Transactions on Knowledge and Data Engineering.
Jiang, B., Liu, Y., and Chan, W. K. (2018). Contractfuzzer: Fuzzing smart contracts for vulnerability detection. In Proceedings of the 33rd ACM/IEEE international conference on automated software engineering, pages 259–269.
Jiang, H., Pei, J., Yu, D., Yu, J., Gong, B., and Cheng, X. (2021). Applications of differential privacy in social network analysis: A survey. IEEE transactions on knowledge and data engineering, 35(1):108–127.
Jing, L. e Tian, Y. (2020). Self-supervised visual feature learning with deep neural networks: A survey. IEEE transactions on pattern analysis and machine intelligence, 43(11):4037–4058.
Jorgensen, Z., Yu, T., and Cormode, G. (2016). Publishing attributed social graphs with formal privacy guarantees. In Proceedings of the 2016 international conference on management of data, pages 107–122.
Juliano, A. (2018). dydx: A standard for decentralized margin trading and derivatives. URl: [link].
Kalra, S., Goel, S., Dhawan, M., and Sharma, S. (2018). Zeus: analyzing safety of smart contracts. In Ndss, pages 1–12.
Karwa, V. and Slavkovi´c, A. B. (2012). Differentially private graphical degree sequences and synthetic graphs. In International Conference on Privacy in Statistical Databases, pages 273–285. Springer.
Karwa, V., Raskhodnikova, S., Smith, A., and Yaroslavtsev, G. (2011). Private analysis of graph structure. PVLDB, 4(11):1146–1157.
Kasiviswanathan, S. P., Nissim, K., Raskhodnikova, S., and Smith, A. (2013). Analyzing graphs with node differential privacy. In Theory of Cryptography Conference, pages 457–476. Springer.
Kaytoue, M., Plantevit, M., Zimmermann, A., Bendimerad, A., and Robardet, C. (2017). Exceptional contextual subgraph mining. Machine Learning, 106:1171–1211.
Kenthapadi, K., Mironov, I., and Thakurta, A. (2019). Privacypreserving data mining in industry. In Companion Proceedings of The 2019 World Wide Web Conference, pages 1308–1310. ACM.
Kim, D., Cho, D., Yoo, D. e Kweon, I. S. (2018). Learning image representations by completing damaged jigsaw puzzles. Em 2018 IEEE Winter Conference on Applications of Computer Vision (WACV), p. 793–802. IEEE.
Kossinets, G. and Watts, D. J. (2006). Empirical analysis of an evolving social network. science, 311(5757):88–90.
Krizhevsky, A., Sutskever, I. e Hinton, G. E. (2012). Imagenet classification with deep convolutional neural networks. Advances in neural information processing systems, 25.
Kushwaha, S. S., Joshi, S., Singh, D., Kaur, M., and Lee, H.-N. (2022). Ethereum smart contract analysis tools: A systematic review. Ieee Access, 10:57037–57062.
Kwon, J. and Buchman, E. (2019). Cosmos whitepaper. A Netw. Distrib. Ledgers, 27:1–32.
Kye, H., Kim, M. e Kwon, M. (2022). Hierarchical detection of network anomalies: A self-supervised learning approach. IEEE Signal Processing Letters, 29:1908–1912.
Laeuchli, J., Ramírez-Cruz, Y., and Trujillo-Rasua, R. (2022). Analysis of centrality measures under differential privacy models. Applied Mathematics and Computation, 412:126546.
Lamport, L., Shostak, R., and Pease, M. (2019). The Byzantine Generals Problem, page 203–226. Association for Computing Machinery, New York, NY, USA.
Lan, Z., Chen, M., Goodman, S., Gimpel, K., Sharma, P. e Soricut, R. (2019). Albert: A lite bert for self-supervised learning of language representations. arXiv preprint arXiv:1909.11942.
Lazoti, R. (2016). Armazenando dados com Redis. Casa do Código.
Le-Khac, P. H., Healy, G. e Smeaton, A. F. (2020). Contrastive representation learning: A framework and review. IEEE Access, 8:193907–193934.
Lee, P., Bubeck, S. e Petro, J. (2023). Benefits, limits, and risks of gpt-4 as an ai chatbot for medicine.
Lerner, S. D., Cid-Fuentes, J. Á., Len, J., Fernàndez-València, R., Gallardo, P., Vescovo, N., Laprida, R., Mishra, S., Jinich, F., and Masini, D. (2022). Rsk: A bitcoin sidechain with stateful smart-contracts. Cryptology ePrint Archive.
Li, C., Yang, J., Zhang, P., Gao, M., Xiao, B., Dai, X., Yuan, L. e Gao, J. (2021). Efficient self-supervised vision transformers for representation learning. arXiv preprint arXiv:2106.09785.
Li, L., Lu, Y., Yang, G. e Yan, X. (2024a). End-to-end network intrusion detection based on contrastive learning. Sensors, 24(7).
Li, L., Zhao, Y., Luo, S., Wang, G., and Wang, Z. (2023). Efficient community search in edge-attributed graphs. IEEE Transactions on Knowledge and Data Engineering.
Li, M., Weng, J., Li, Y., Wu, Y., Weng, J., Li, D., Xu, G., and Deng, R. (2021). Ivycross: A privacy-preserving and concurrency control framework for blockchain interoperability. Cryptology ePrint Archive.
Li, N., Li, T., and Venkatasubramanian, S. (2006). t-closeness: Privacy beyond k-anonymity and l-diversity. In 2007 IEEE 23rd international conference on data engineering, pages 106–115. IEEE.
Li, N., Lyu, M., Su, D., and Yang, W. (2016). Differential privacy: From theory to practice. Synthesis Lectures on Information Security, Privacy, & Trust, 8(4):1–138.
Li, P., Li, S., Ding, M., Yu, J., Zhang, H., Zhou, X., and Li, J. (2022). A vulnerability detection framework for hyperledger fabric smart contracts based on dynamic and static analysis. In Proceedings of the 26th International Conference on Evaluation and Assessment in Software Engineering, pages 366–374.
Li, X., Yang, J., Sun, Z., and Zhang, J. (2017). Differential privacy for edge weights in social networks. Security and Communication Networks, 2017.
Li, Z., Xia, L., Xu, Y. e Huang, C. (2024b). Gpt-st: Generative pretraining of spatio-temporal graph neural networks. Advances in Neural Information Processing Systems, 36.
Liao, Z., Zheng, Z., Chen, X., and Nan, Y. (2022). Smartdagger: a bytecode-based static analysis approach for detecting cross-contract vulnerability. In Proceedings of the 31st ACM SIGSOFT International Symposium on Software Testing and Analysis, pages 752–764.
Linoy, S., Ray, S., and Stakhanova, N. (2021). Etherprov: Provenanceaware detection, analysis, and mitigation of ethereum smart contract security issues. In 2021 IEEE International Conference on Blockchain (Blockchain), pages 1–10. IEEE.
Liu, H., Li, S., Li, H., Li, X., Gao, J. e Ji, S. (2019a). Graphaf: A flow-based autoregressive model for molecular graph generation. Em Proceedings of the 36th International Conference on Machine Learning, p. 3872–3881.
Liu, X., Zhang, F., Hou, Z., Mian, L., Wang, Z., Zhang, J. e Tang, J. (2023). Self-supervised learning: Generative or contrastive. IEEE Transactions on Knowledge and Data Engineering, 35(1):857–876.
Liu, Y., Ott, M., Goyal, N., Du, J., Joshi, M., Chen, D., Levy, O., Lewis, M., Zettlemoyer, L. e Stoyanov, V. (2019b). Roberta: A robustly optimized bert pretraining approach. arXiv preprint arXiv:1907.11692.
Liu, Z., Huang, L., Xu, H., Yang, W., and Wang, S. (2020). Privag: Analyzing attributed graph data with local differential privacy. In 2020 IEEE 26th International Conference on Parallel and Distributed Systems (ICPADS), pages 422–429. IEEE.
Liu, Z., Qian, P., Yang, J., Liu, L., Xu, X., He, Q., and Zhang, X. (2023). Rethinking smart contract fuzzing: Fuzzing with invocation ordering and important branch revisiting. IEEE Transactions on Information Forensics and Security, 18:1237–1251.
Lopez-Martin, M., Sanchez-Esguevillas, A., Arribas, J. I. e Carro, B. (2023). Contrastive learning over random fourier features for iot network intrusion detection. IEEE Internet of Things Journal, 10(10):8505–8513.
Luu, L., Chu, D.-H., Olickel, H., Saxena, P., and Hobor, A. (2016). Making smart contracts smarter. In Proceedings of the 2016 ACM SIGSAC conference on computer and communications security, pages 254–269.
Ma, C., Kong, X., Lan, Q., and Zhou, Z. (2019). The privacy protection mechanism of hyperledger fabric and its application in supply chain finance. Cybersecurity, 2(1):1–9.
Machanavajjhala, A., Kifer, D., Gehrke, J., and Venkitasubramaniam, M. (2007). l-diversity: Privacy beyond k-anonymity. ACM Transactions on Knowledge Discovery from Data (TKDD), 1(1):3–es.
Magalhães, A., Brayner, Â., and Monteiro, J. M. (2023). Bancos de dados em memória e suas estratégias de recuperação após falhas. Sociedade Brasileira de Computação.
Magalhães, A., Brayner, A., and Monteiro, J. M. (2023a). Main memory database instant recovery. In Anais Estendidos do XXXVIII Simpósio Brasileiro de Bancos de Dados, pages 255–269. SBC.
Magalhães, A., Brayner, A., and Monteiro, J. M. (2023b). Main memory database recovery strategies. In Das, S., Pandis, I., Candan, K. S., and Amer-Yahia, S., editors, Companion of the 2023 International Conference on Management of Data, SIGMOD/PODS 2023, Seattle, WA, USA, June 18-23, 2023, pages 31–35. ACM.
Magalhães, A., Monteiro, J. M., and Brayner, A. (2018). Gerenciamento e processamento de big data com bancos de dados em memória. In I Jornada latino-americana de atualização em informática , JOLAI 2017, São Paulo, SP, Brazil, 2018.
Magalhães, A., Monteiro, J. M., and Brayner, A. (2021). Main memory database recovery: A survey. ACM Comput. Surv., 54(2):46:1–46:36.
Mahadevan, P., Krioukov, D., Fall, K., and Vahdat, A. (2006). Systematic topology analysis and generation using degree correlations. ACM SIGCOMM Computer Communication Review, 36(4):135–146.
Marquesone, R. (2016). Big Data: Técnicas e tecnologias para extração de valor dos dados. Editora Casa do Código.
McMahan, B., Moore, E., Ramage, D., Hampson, S. e y Arcas, B. A. (2017). Communication-efficient learning of deep networks from decentralized data. Em Artificial intelligence and statistics, p. 1273–1282. PMLR.
McPherson, M., Smith-Lovin, L., and Cook, J. M. (2001). Birds of a feather: Homophily in social networks. Annual review of sociology, 27(1):415–444.
McSherry, F. and Talwar, K. (2007). Mechanism design via differential privacy. In 48th Annual IEEE Symposium on Foundations of Computer Science, pages 94–103. IEEE.
Mendonça, A. L., Brito, F. T., and Machado, J. C. (2023). Privacy-preserving techniques for social network analysis. In Anais Estendidos do XXXVIII Simpósio Brasileiro de Bancos de Dados, pages 174–178. SBC.
Mendonça, R. D., Cardoso, I. W. F., Coelho, R., Campos, J. N., Gonçalves, G. D., Vieira, A. B., and Nacif, J. A. (2024). Mecanismos de interoperabilidade em blockchains: Um comparativo de custo de transações cross-chain para tokens erc-20. In Anais do VII Workshop em Blockchain: Teoria, Tecnologias e Aplicações. SBC.
Messias, J., Pahari, V., Chandrasekaran, B., Gummadi, K. P., and Loiseau, P. (2023). Understanding blockchain governance: Analyzing decentralized voting to amend defi smart contracts.
Michael, M. M., Moreira, J. E., Shiloach, D., and Wisniewski, R. W. (2007). Scale-up x scale-out: A case study using nutch/lucene. In 21th International Parallel and Distributed Processing Symposium (IPDPS 2007), Proceedings, 26-30 March 2007, Long Beach, California, USA, pages 1–8. IEEE.
Mikolov, T., Sutskever, I., Chen, K., Corrado, G. S. e Dean, J. (2013). Distributed representations of words and phrases and their compositionality. Advances in neural information processing systems, 26.
Mislove, A., Viswanath, B., Gummadi, K. P., and Druschel, P. (2010). You are who you know: inferring user profiles in online social networks. In Proceedings of the third ACM international conference on Web search and data mining, pages 251–260.
Misra, I. e Maaten, L. v. d. (2020). Self-supervised learning of pretext-invariant representations. Em Proceedings of the IEEE/CVF conference on computer vision and pattern recognition, p. 6707–6717.
Mitchell, J. S. (2017). Shortest paths and networks. In Handbook of discrete and computational geometry, pages 811–848. Chapman and Hall/CRC.
Mohamed, M. S., Nguyen, D., Vullikanti, A., and Tandon, R. (2022). Differentially private community detection for stochastic block models. In International Conference on Machine Learning, pages 15858–15894. PMLR.
Mohammad, S. (2016). Self-tuning for cloud database clusters. PhD thesis, University of Magdeburg, Germany.
MongoDB Database (2024). MongoDB: The Developer Data Platform | MongoDB. Disponível em: [link]. Acessado em: 01/05/2024.
MongoDB Documentation (2024). Mongodb documentation. Disponível em: [link]. Acessado em: 01/05/2024.
Mossberg, M., Manzano, F., Hennenfent, E., Groce, A., Grieco, G., Feist, J., Brunson, T., and Dinaburg, A. (2019). Manticore: A user-friendly symbolic execution framework for binaries and smart contracts. In 2019 34th IEEE/ACM (ASE), pages 1186–1189. IEEE.
Mueller, T. T., Paetzold, J. C., Prabhakar, C., Usynin, D., Rueckert, D., and Kaissis, G. (2022). Differentially private graph neural networks for wholegraph classification. IEEE Transactions on Pattern Analysis and Machine Intelligence.
Murray, A., Kim, D., and Combs, J. (2023). The promise of a decentralized internet: What is web3 and how can firms prepare? Business Horizons, 66(2):191–202.
Nadini, M., Alessandretti, L., Di Giacinto, F., Martino, M., Aiello, L. M., and Baronchelli, A. (2021). Mapping the nft revolution: market trends, trade networks, and visual features. Scientific reports, 11(1):1–11.
Nakamoto, S. (2008). Bitcoin: A peer-to-peer electronic cash system.
Nakamoto, S. and Bitcoin, A. (2008). A peer-to-peer electronic cash system. Bitcoin.–URL: [link]. pdf, 4.
Narayanan, A. and Shmatikov, V. (2008). Robust deanonymization of large sparse datasets. In 2008 IEEE Symposium on Security and Privacy (sp 2008), pages 111–125. IEEE.
Narayanan, A. and Shmatikov, V. (2009). Deanonymizing social networks. In Security and Privacy, 2009 30th IEEE Symposium on, pages 173–187. IEEE.
Near, J. P. and Abuah, C. (2021). Programming differential privacy. URL: [link].
Neo4J Database (2024). Neo4j Graph Database & Analytics. Disponível em: [link]. Acessado em: 01/05/2024.
Neo4J Documentation (2024). Neo4j documentation. Disponível em: [link]. Acessado em: 01/05/2024.
Nergiz, M. E., Atzori, M., and Clifton, C. (2007). Hiding the presence of individuals from shared databases. In Proceedings of the 2007 ACM SIGMOD International Conference on Management of Data, pages 665–676.
Nguyen, H. H., Imine, A., and Rusinowitch, M. (2015). Differentially private publication of social graphs at linear cost. In 2015 IEEE/ACM International Conference on Advances in Social Networks Analysis and Mining (ASONAM), pages 596–599. IEEE.
Nguyen, H. H., Imine, A., and Rusinowitch, M. (2016). Detecting communities under differential privacy. In Proceedings of the 2016 ACM on Workshop on Privacy in the Electronic Society, pages 83–93.
Nissim, K., Raskhodnikova, S., and Smith, A. (2007). Smooth sensitivity and sampling in private data analysis. In Proceedings of the thirty-ninth annual ACM symposium on Theory of computing, pages 75–84.
Noroozi, M. e Favaro, P. (2016). Unsupervised learning of visual representations by solving jigsaw puzzles. Em European conference on computer vision, p. 69–84. Springer.
Okonkwo, I. E. (2021). Nft, copyright; and intellectual property commercialisation. SSRN. [link].
Ongaro, D. and Ousterhout, J. (2014). In search of an understandable consensus algorithm. In 2014 USENIX annual technical conference (USENIX ATC 14), pages 305–319.
Oord, A. v. d., Dieleman, S., Zen, H., Simonyan, K., Vinyals, O., Graves, A., Kalchbrenner, N., Senior, A. e Kavukcuoglu, K. (2016a). Wavenet: A generative model for raw audio. arXiv preprint arXiv:1609.03499.
Oord, A. v. d., Kalchbrenner, N. e Kavukcuoglu, K. (2016b). Pixel recurrent neural networks. arXiv preprint arXiv:1601.06759.
Oord, A. v. d., Li, Y. e Vinyals, O. (2018). Representation learning with contrastive predictive coding. arXiv preprint arXiv:1807.03748.
Ou, W., Huang, S., Zheng, J., Zhang, Q., Zeng, G., and Han, W. (2022). An overview on cross-chain: Mechanism, platforms, challenges and advances. Computer Networks.
Özsu, M. T. and Valduriez, P. (1996). Distributed and parallel database systems. ACM Comput. Surv., 28(1):125–128.
Palma, L., Martina, J., and Vigil, M. (2022). On and off: Extracting the transaction history of permissioned blockchain networks. Universidade Federal de Santa Catarina. Computing Science PhD Candidate Dissertation.
Paniz, D. (2016). NoSQL: Como armazenar os dados de uma aplicação moderna. Casa do Código.
Passos, P. V., de Oliveira, L. S., Schreiner, G. A., Machado, V. L., and dos Santos Mello, R. (2023). Sql2neo: Uma interface de acesso SQL para o neo4j. In Proceedings of the 38th Brazilian Symposium on Databases, SBBD 2023, Belo Horizonte, MG, Brazil, September 25-29, 2023, pages 179–191. SBC.
Peng, J., Liu, D., Xu, S. e Li, H. (2021). Generating diverse structure for image inpainting with hierarchical vq-vae. Em Proceedings of the IEEE/CVF Conference on Computer Vision and Pattern Recognition, p. 10775–10784.
Perozzi, B., Al-Rfou, R. e Skiena, S. (2014). Deepwalk: Online learning of social representations. Em Proceedings of the 20th ACM SIGKDD international conference on Knowledge discovery and data mining, p. 701–710. ACM.
Phillips, D. J., McGlaughlin, A., Ruth, D., Jager, L. R., and Soldan, A. (2015). Graph theoretic analysis of structural connectivity across the spectrum of Alzheimer’s disease: The importance of graph creation methods. NeuroImage: Clinical, 7:377–390.
Pinot, R., Morvan, A., Yger, F., Gouy-Pailler, C., and Atif, J. (2018). Graph-based clustering under differential privacy. arXiv preprint arXiv:1803.03831.
Popova, M., Shvets, M., Oliva, J. e Isayev, O. (2019). Molecularrnn: Generating realistic molecular graphs with optimized properties. arXiv preprint arXiv:1905.13372.
Prell, C. (2011). Social network analysis: History, theory and methodology. Sage.
Product (2024). dYdX v4 - full decentralization. [link]. (Accessed on 05/20/2024).
Qian, J., Li, X.-Y., Zhang, C., and Chen, L. (2016). De-anonymizing social networks and inferring private attributes using knowledge graphs. In IEEE INFOCOM 2016-The 35th Annual IEEE International Conference on Computer Communications, pages 1–9. IEEE.
Qin, K., Zhou, L., and Gervais, A. (2021). Quantifying blockchain extractable value: How dark is the forest? CoRR, abs/2101.05511.
Ragab, M., Eldele, E., Chen, Z., Wu, M., Kwoh, C.-K. e Li, X. (2022). Self-supervised autoregressive domain adaptation for time series data. IEEE Transactions on Neural Networks and Learning Systems.
Ramakrishnan, R. and Gehrke, J. (2003). Database management systems (3. ed.). McGraw-Hill.
Razavi, A., Van den Oord, A. e Vinyals, O. (2019). Generating diverse high-fidelity images with vq-vae-2. Advances in neural information processing systems, 32.
Redis Database (2024). Redis - the real-time data platform. Disponível em: [link]. Acessado em: 01/05/2024.
Redis Documentation (2024). Redis documentation! Disponível em: [link]. Acessado em: 01/05/2024.
Redis Insight (2024). Redis insight the best redis gui. Disponível em: [link]. Acessado em: 01/05/2024.
Ren, K., Ho, N.-M., Loghin, D., Nguyen, T.-T., Ooi, B. C., Ta, Q.-T., and Zhu, F. (2023). Interoperability in blockchain: A survey. IEEE Transactions on Knowledge and Data Engineering.
Ribeiro, P., Paredes, P., Silva, M. E., Aparicio, D., and Silva, F. (2021). A survey on subgraph counting: concepts, algorithms, and applications to network motifs and graphlets. ACM Computing Surveys (CSUR), 54(2):1–36.
Rodler, M., Paaßen, D., Li, W., Bernhard, L., Holz, T., Karame, G., and Davi, L. (2023). Ef cf: High performance smart contract fuzzing for exploit generation. In 2023 IEEE 8th European Symposium on Security and Privacy (EuroS&P), pages 449–471. IEEE.
Rodrigues, W. B. et al. (2017). Nosql: a análise da modelagem e consistência dos dados na era do big data.
Roohi, L., Rubinstein, B. I., and Teague, V. (2019). Differentiallyprivate two-party egocentric betweenness centrality. In IEEE INFOCOM 2019-IEEE Conference on Computer Communications, pages 2233–2241. IEEE.
Rouhani, S. and Deters, R. (2019). Security, performance, and applications of smart contracts: A systematic survey. IEEE Access, 7:50759–50779.
Sabt, M., Achemlal, M., and Bouabdallah, A. (2015). Trusted execution environment: What it is, and what it is not. In 2015 IEEE Trustcom/BigDataSE/Ispa, volume 1, pages 57–64. IEEE.
Sadalage, P. J. and Fowler, M. (2019). NoSQL essencial: um guia conciso para o mundo emergente da persistência poliglota. Novatec Editora.
Sala, A., Zhao, X., Wilson, C., Zheng, H., and Zhao, B. Y. (2011). Sharing graphs using differentially private graph models. In Proceedings of the 2011 ACM SIGCOMM conference on Internet measurement conference, pages 81–98.
Samoylenko, I., Aleja, D., Primo, E., Alfaro-Bittner, K., Vasilyeva, E., Kovalenko, K., Musatov, D., Raigorodskii, A. M., Criado, R., Romance, M., et al. (2023). Why are there six degrees of separation in a social network? Physical Review X, 13(2):021032.
Sanches, B. H. and Diniz, E. (2024). From crypto-libertarian utopia to central bank digital currencies: The transfigurative convergence of bitcoin’s prefiguration. Available at SSRN 4818428.
Sayeed, S., Marco-Gisbert, H., and Caira, T. (2020). Smart contract: Attacks and protections. IEEE Access, 8:24416–24427.
Schär, F. (2021). Decentralized finance: On blockchain-and smart contractbased financial markets. FRB of St. Louis Review.
Sealfon, A. (2016). Shortest paths and distances with differential privacy. In Proceedings of the 35th Symposium on Principles of Database Systems, pages 29–41.
Shah, N., Beutel, A., Hooi, B., Akoglu, L., Gunnemann, S., Makhija, D., Kumar, M., and Faloutsos, C. (2016). Edgecentric: Anomaly detection in edgeattributed networks. In 2016 IEEE 16Th international conference on data mining workshops (ICDMW), pages 327–334. IEEE.
Shi, C., Li, Y., Zhang, J., Sun, Y., and Philip, S. Y. (2016). A survey of heterogeneous information network analysis. IEEE Transactions on Knowledge and Data Engineering, 29(1):17–37.
Silva, L. F. C., Riva, A. D., and Rosa, G. A. (2021). Banco de Dados Não Relacional. Minha Biblioteca.
Silva, R. R. C., Leal, B. C., Brito, F. T., Vidal, V. M., and Machado, J. C. (2017). A differentially private approach for querying rdf data of social networks. In Proceedings of the 21st International Database Engineering & Applications Symposium, pages 74–81.
Singh, A., Click, K., Parizi, R. M., Zhang, Q., Dehghantanha, A., and Choo, K.-K. R. (2020). Sidechain technologies in blockchain networks: An examination and state-of-the-art review. Journal of Network and Computer Applications, 149:102471.
Siris, V. A., Nikander, P., Voulgaris, S., Fotiou, N., Lagutin, D., and Polyzos, G. C. (2019). Interledger approaches. Ieee Access, 7:89948–89966.
Song, K., Matulevicius, N., de Lima Filho, E. B., and Cordeiro, L. C. (2022). Esbmc-solidity: An smt-based model checker for solidity smart contracts. In Proceedings of the ACM/IEEE 44th International Conference on Software Engineering: Companion Proceedings, pages 65–69.
Stafford, V. (2020). Zero trust architecture. NIST special publication, 800:207.
Strauch, C., Sites, U.-L. S., and Kriha, W. (2011). Nosql databases. Lecture Notes, Stuttgart Media University, 20(24):79.
Studio 3T (2024). Studio 3T, the Ultimate GUI for MongoDB. Disponível em: [link]. Acessado em: 01/05/2024.
Sun, H., Xiao, X., Khalil, I., Yang, Y., Qin, Z., Wang, H., and Yu, T. (2019). Analyzing subgraph statistics from extended local views with decentralized differential privacy. In Proceedings of the 2019 ACM SIGSAC Conference on Computer and Communications Security, pages 703–717.
Sun, X., Yu, F. R., Zhang, P., Sun, Z., Xie, W., and Peng, X. (2021). A survey on zero-knowledge proof in blockchain. IEEE network, 35(4):198–205.
Sweeney, L. (2002). k-anonymity: A model for protecting privacy. International Journal of Uncertainty, Fuzziness and Knowledge-Based Systems, 10(05):557–570.
Szabo, N. (1997). Formalizing and securing relationships on public networks. First monday.
Taft, R., Mansour, E., Serafini, M., Duggan, J., Elmore, A. J., Aboulnaga, A., Pavlo, A., and Stonebraker, M. (2014). E-store: Fine-grained elastic partitioning for distributed transaction processing. Proceedings of the VLDB Endowment, 8(3):245–256.
Tang, J., Qu, M., Wang, M., Zhang, M., Yan, J. e Mei, Q. (2015). Line: Large-scale information network embedding. Em Proceedings of the 24th international conference on world wide web, p. 1067–1077.
Task, C. and Clifton, C. (2014). What should we protect? defining differential privacy for social network analysis. In State of the Art Applications of Social Network Analysis, pages 139–161. Springer.
Teixeira, D. (2023). O caminho para o real digital. Revista LIFT papers, 5(5).
Thisanke, H., Deshan, C., Chamith, K., Seneviratne, S., Vidanaarachchi, R. e Herath, D. (2023). Semantic segmentation using vision transformers: A survey. Engineering Applications of Artificial Intelligence, 126:106669.
Thomas, S. and Schwartz, E. (2015). A protocol for interledger payments. URL [link].
Tian, S., Gao, Y., Yuan, G., Zhang, R., Zhao, J. e Zhang, S. (2022). An encrypted traffic classification method based on contrastive learning. Em Proceedings of the 8th International Conference on Communication and Information Processing, p. 101–105.
Tian, Y., Krishnan, D. e Isola, P. (2020). Contrastive multiview coding. Em Computer Vision–ECCV 2020: 16th European Conference, Glasgow, UK, August 23–28, 2020, Proceedings, Part XI 16, p. 776–794. Springer.
Tikhomirov, S. (2018). Ethereum: state of knowledge and research perspectives. In Foundations and Practice of Security: 10th International Symposium, FPS 2017, Nancy, France, October 23-25, 2017, Revised Selected Papers 10, pages 206–221. Springer.
Tikhomirov, S., Voskresenskaya, E., Ivanitskiy, I., Takhaviev, R., Marchenko, E., and Alexandrov, Y. (2018). Smartcheck: Static analysis of ethereum smart contracts. In Proceedings of the 1st international workshop on emerging trends in software engineering for blockchain, pages 9–16.
Torbarina, L., Ferkovic, T., Roguski, L., Mihelcic, V., Sarlija, B. e Kraljevic, Z. (2024). Challenges and opportunities of using transformer-based multi-task learning in nlp through ml lifecycle: A position paper. Natural Language Processing Journal, 7:100076.
Torres, C. F., Camino, R., and State, R. (2021). Frontrunner jones and the raiders of the dark forest: An empirical study of frontrunning on the ethereum blockchain. In 30th USENIX Security Symposium (USENIX Security 21), pages 1343–1359. USENIX Association.
Van den Oord, A., Kalchbrenner, N., Espeholt, L., Vinyals, O., Graves, A. et al. (2016). Conditional image generation with pixelcnn decoders. Advances in neural information processing systems, 29.
Varun, M., Palanisamy, B., and Sural, S. (2022). Mitigating frontrunning attacks in ethereum. In Proceedings of the Fourth ACM International Symposium on Blockchain and Secure Critical Infrastructure, BSCI ’22, page 115–124. Association for Computing Machinery.
Vaswani, A., Shazeer, N., Parmar, N., Uszkoreit, J., Jones, L., Gomez, A. N., Kaiser, Ł. e Polosukhin, I. (2017). Attention is all you need. Advances in neural information processing systems, 30.
Vieira, M. R., FIGUEIREDO, J. M. d., Liberatti, G., and Viebrantz, A. F. M. (2012). Bancos de dados nosql: conceitos, ferramentas, linguagens e estudos de casos no contexto de big data. Simpósio Brasileiro de Bancos de Dados, 27:1–30.
VolDB Documentation (2020). Voldb documentation. Disponível em: [link]. Acessado em: 01/05/2024.
Wang, C.-D., Lai, J.-H., and Philip, S. Y. (2013). Neiwalk: Community discovery in dynamic content-based networks. IEEE transactions on knowledge and data engineering, 26(7):1734–1748.
Wang, D. and Long, S. (2019). Boosting the accuracy of differentially private in weighted social networks. Multimedia Tools and Applications, 78(24):34801–34817.
Wang, D., Wu, S., Lin, Z., Wu, L., Yuan, X., Zhou, Y., Wang, H., and Ren, K. (2021). Towards a first step to understand flash loan and its applications in defi ecosystem. In Proceedings of the Ninth International Workshop on Security in Blockchain and Cloud Computing, pages 23–28.
Wang, N., Chen, Y., Hu, Y., Lou, W. e Hou, Y. T. (2022a). Feco: Boosting intrusion detection capability in iot networks via contrastive learning. Em IEEE INFOCOM 2022-IEEE Conference on Computer Communications, p. 1409–1418. IEEE.
Wang, T., Blocki, J., Li, N., and Jha, S. (2017). Locally differentially private protocols for frequency estimation. In 26th USENIX Security Symposium (USENIX Security 17), pages 729–745.
Wang, W., Song, J., Xu, G., Li, Y., Wang, H., and Su, C. (2020). Contractward: Automated vulnerability detection models for ethereum smart contracts. IEEE Transactions on Network Science and Engineering.
Wang, X., Xu, H., Wang, X., Xu, X. e Wang, Z. (2022b). A graph neural network and pointer network-based approach for qos-aware service composition. IEEE Transactions on Services Computing.
Wang, Y. and Wu, X. (2013). Preserving differential privacy in degree-correlation based graph generation. Transactions on data privacy, 6(2):127.
Wang, Y., Zuest, P., Yao, Y., Lu, Z., andWattenhofer, R. (2022). Impact and user perception of sandwich attacks in the defi ecosystem. In Proceedings of the 2022 CHI Conference on Human Factors in Computing Systems, CHI ’22. Association for Computing Machinery.
Wegner, P. (1996). Interoperability. ACM Computing Surveys (CSUR), 28(1):285–287.
Wei, C., Xie, L., Ren, X., Xia, Y., Su, C., Liu, J., Tian, Q. e Yuille, A. L. (2019). Iterative reorganization with weak spatial constraints: Solving arbitrary jigsaw puzzles for unsupervised representation learning. Em Proceedings of the IEEE/CVF Conference on Computer Vision and Pattern Recognition, p. 1910–1919.
Weintraub, B., Torres, C. F., Nita-Rotaru, C., and State, R. (2022). A flash(bot) in the pan: measuring maximal extractable value in private pools. In Proceedings of the 22nd ACM Internet Measurement Conference, IMC ’22, page 458–471. Association for Computing Machinery.
Wen, L., Li, X. e Gao, L. (2020). A transfer convolutional neural network for fault diagnosis based on resnet-50. Neural Computing and Applications, 32(10):6111–6124.
Werner, S., Perez, D., Gudgeon, L., Klages-Mundt, A., Harz, D., and Knottenbelt, W. (2022). Sok: Decentralized finance (defi). In Proceedings of the 4th ACM Conference on Advances in Financial Technologies, pages 30–46.
White, B., Mahanti, A., and Passi, K. (2022). Characterizing the opensea nft marketplace. In Companion Proceedings of the Web Conference 2022, pages 488–496.
White, G., Palade, A., Cabrera, C. e Clarke, S. (2019). Autoencoders for qos prediction at the edge. Em 2019 IEEE International Conference on Pervasive Computing and Communications (PerCom, p. 1–9.
Wood, G. (2014). Ethereum: A secure decentralised generalised transaction ledger. Ethereum project yellow paper, 151:1–32.
Wu, L., Lin, H., Tan, C., Gao, Z. e Li, S. Z. (2023). Self-supervised learning on graphs: Contrastive, generative, or predictive. IEEE Transactions on Knowledge and Data Engineering, 35(4):4216–4235.
Wu, S., Wang, D., He, J., Zhou, Y., Wu, L., Yuan, X., He, Q., and Ren, K. (2021). Defiranger: Detecting price manipulation attacks on defi applications. arXiv preprint arXiv:2104.15068.
Wüstholz, V. and Christakis, M. (2020). Harvey: A greybox fuzzer for smart contracts. In Proceedings of the 28th ACM Joint Meeting on European Software Engineering Conference and Symposium on the Foundations of Software Engineering, pages 1398–1409.
Xiao, Q., Chen, R., and Tan, K.-L. (2014). Differentially private network data release via structural inference. In Proceedings of the 20th ACM SIGKDD international conference on Knowledge discovery and data mining, pages 911–920.
Xu, X., Hu, T., Li, B., and Liao, L. (2023). Ccdetector: Detect chaincode vulnerabilities based on knowledge graph. In 2023 IEEE 47th Annual Computers, Software, and Applications Conference (COMPSAC), pages 699–704. IEEE.
Xu, X., Weber, I., and Staples, M. (2019). Architecture for blockchain applications. Springer.
Xu, Y., Hu, G., You, L., and Cao, C. (2021). A novel machine learningbased analysis model for smart contract vulnerability. Security and Communication Networks, 2021:1–12.
Yadav, K. and Naval, S. (2023). Cfg analysis for detecting vulnerabilities in smart contracts. In International Conference on Smart Trends for Information Technology and Computer Communications, pages 753–763. Springer.
Yamashita, K., Nomura, Y., Zhou, E., Pi, B., and Jun, S. (2019). Potential risks of hyperledger fabric smart contracts. In 2019 IEEE International Workshop on Blockchain Oriented Software Engineering (IWBOSE), pages 1–10. IEEE.
Yan, X., Wang, S., and Gai, K. (2022). A semantic analysis-based method for smart contract vulnerability. In 2022 IEEE 8th Intl Conference on Big Data Security on Cloud (BigDataSecurity), pages 23–28. IEEE.
Yang, S., Wang, Y., Xu, H., Xu, F. e Chen, M. (2022). An android malware detection and classification approach based on contrastive lerning. Computers & Security, 123:102915.
Yang, Z., Dai, Z., Yang, Y., Carbonell, J., Salakhutdinov, R. R. e Le, Q. V. (2019). Xlnet: Generalized autoregressive pretraining for language understanding. Advances in neural information processing systems, 32.
Ye, J., Ma, M., Lin, Y., Sui, Y., and Xue, Y. (2020). Clairvoyance: Cross-contract static analysis for detecting practical reentrancy vulnerabilities in smart contracts. In Proceedings of the ACM/IEEE 42nd International Conference on Software Engineering: Companion Proceedings, pages 274–275.
Ye, M. and Barg, A. (2018). Optimal schemes for discrete distribution estimation under locally differential privacy. IEEE Transactions on Information Theory, 64(8):5662–5676.
Yin, Y., Di, Q., Wan, J. e Liang, T. (2023). Time-aware smart city services based on qos prediction: A contrastive learning approach. IEEE Internet of Things Journal.
You, J., Liu, B., Ying, Z., Pande, V. e Leskovec, J. (2018a). Graph convolutional policy network for goal-directed molecular graph generation. Advances in neural information processing systems, 31.
You, J., Ying, R., Ren, X., Hamilton, W. e Leskovec, J. (2018b). Graphrnn: Generating realistic graphs with deep auto-regressive models. Em International Conference on Machine Learning, p. 5708–5717.
You, Y., Gitman, I. e Ginsburg, B. (2017). Large batch training of convolutional networks. arXiv preprint arXiv:1708.03888.
Yue, Y., Chen, X., Han, Z., Zeng, X. e Zhu, Y. (2022). Contrastive learning enhanced intrusion detection. IEEE Transactions on Network and Service Management, 19(4):4232–4247.
Zafar, S., Lv, Z., Zaydi, N. H., Ibrar, M. e Hu, X. (2022). Dsmlb: Dynamic switch-migration based load balancing for software-defined iot network. Computer Networks, 214:109145.
Zbontar, J., Jing, L., Misra, I., LeCun, Y. e Deny, S. (2021). Barlow twins: Self-supervised learning via redundancy reduction. Em International conference on machine learning, p. 12310–12320. PMLR.
Zeller, A., Gopinath, R., Böhme, M., Fraser, G., and Holler, C. (2019). The fuzzing book.
Zhang, J., Cormode, G., Procopiuc, C. M., Srivastava, D., and Xiao, X. (2015). Private release of graph statistics using ladder functions. In Proceedings of the 2015 ACM SIGMOD international conference on management of data, pages 731–745.
Zhang, P., Yu, Q., Xiao, Y., Dong, H., Luo, X., Wang, X., and Zhang, M. (2023a). Bian: Smart contract source code obfuscation. IEEE Transactions on Software Engineering.
Zhang, W., Wei, L., Cheung, S.-C., Liu, Y., Li, S., Liu, L., and Lyu, M. R. (2023b). Combatting front-running in smart contracts: Attack mining, benchmark construction and vulnerability detector evaluation. IEEE Transactions on Software Engineering, 49(6):3630–3646.
Zhang, X. e Zhu, Q. (2023). Ai-enabled network-functions virtualization and software-defined architectures for customized statistical qos over 6g massive mimo mobile wireless networks. IEEE Network, 37(2):30–37.
Zhang, X., Zhao, R., Jiang, Z., Sun, Z., Ding, Y., Ngai, E. C. e Yang, S.-H. (2024). Aoc-ids: Autonomous online framework with contrastive learning for intrusion detection. arXiv preprint arXiv:2402.01807.
Zhang, Y., Liu, P., Wang, G., Li, P., Gu, W., Chen, H., Liu, X., and Zhu, J. (2023c). Frad: Front-running attacks detection on ethereum using ternary classification model. arXiv preprint arXiv:2311.14514.
Zhao, W., Xu, G., Cui, Z., Luo, S., Long, C. e Zhang, T. (2023). Deep graph structural infomax. Em Proceedings of the AAAI Conference on Artificial Intelligence, volume 37, p. 4920–4928.
Zheng, Y., Jin, M., Liu, Y., Chi, L., Phan, K. T. e Chen, Y.-P. P. (2021). Generative and contrastive self-supervised learning for graph anomaly detection. IEEE Transactions on Knowledge and Data Engineering.
Zhou, L., Qin, K., Torres, C. F., Le, D. V., and Gervais, A. (2021). High-frequency trading on decentralized on-chain exchanges. In 2021 IEEE Symposium on Security and Privacy (SP), pages 428–445. IEEE.
Zhu, J., Li, B., Wang, J., Li, D., Liu, Y. e Zhang, Z. (2023). Bgcl: Bi-subgraph network based on graph contrastive learning for cold-start qos prediction. Knowledge-Based Systems, 263:110296.
Zhuang, C., Zhai, A. L. e Yamins, D. (2019). Local aggregation for unsupervised learning of visual embeddings. Em Proceedings of the IEEE/CVF international conference on computer vision, p. 6002–6012.
Zoph, B., Cubuk, E. D., Ghiasi, G., Lin, T.-Y., Shlens, J. e Le, Q. V. (2020). Rethinking pre-training and self-training. Em Proceedings of the IEEE/CVF Conference on Computer Vision and Pattern Recognition, p. 10286–10295.
Zou, W., Lo, D., Kochhar, P. S., Le, X.-B. D., Xia, X., Feng, Y., Chen, Z., and Xu, B. (2019). Smart contract development: Challenges and opportunities. IEEE Transactions on Software Engineering.
Zwitter, A. and Hazenberg, J. (2020). Decentralized network governance: blockchain technology and the future of regulation. Frontiers in Blockchain, 3:12.
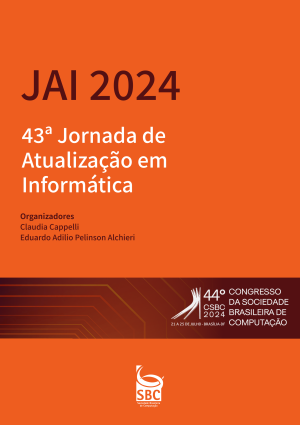
Downloads
Publication date
Series
Categories
License

This work is licensed under a Creative Commons Attribution-NonCommercial 4.0 International License.